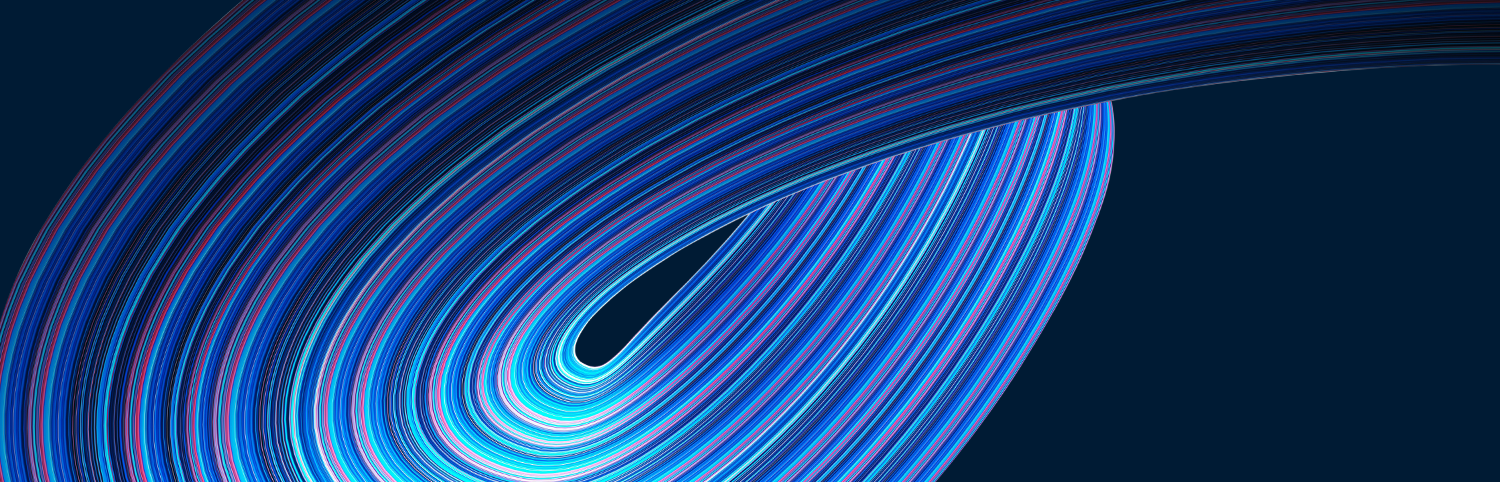
Equal & opposing forces: considerations in AI
By Kent Bhupathi, Group Director, Data Science & Advanced Analytics, SOLVE(D)
SXSW 2023 brought to light not just the growing global obsession with “AI” (mostly through generative modelling technologies), but also our growing dependence. From the text-to-image creation of personal mascots (mine looked like a corporate egghead), the speech-to-video revamp of film dubbing overlays to the hoard of GPT-3+ integrations and plug-ins across software companies, these technologies were everywhere and in all forms!
And while these engagements were incredibly fun and insightful, for each session that discussed the futurists’ point of view, I came across a talk or panel on the pressing need for strict AI governance. But what is “AI governance?” My takeaway, as a definition: it is a rigorous sociotechnical standard that aims to (A) eliminate racial, cultural, sexual and socio-economic bias from training data; and (B) promote the development of modelling forms and outputs that reduce inequalities and inequities.
These types of sessions emphasized that the moral and business imperative for AI governance is more relevant than ever, especially as these models continue to shape our daily lives and while the gatekeepers to the most influential varieties of modelling are laying-off their ethics departments. 1
As a data scientist these sessions grounded me daily—helping to provide a more complete market outlook, not just of what technology can do, but also what all we technologists should do.
Diminish the amplification of algorithmic bias
Generative pre-trained transformers (GPT) algorithms have a significant impact on our digital experiences. At present, many of the paradigms are free for use, and this conceptually allows anyone, from any background, the chance to make use of them. This means that these technologies should be adequately representative of such diversity. However, even if the training set distributionally follows the racial makeup of a region, such as the US (59.3% White, 18.9% Hispanic, 13.6% African American, 6.1% Asian, etc),2 the probabilistic and pattern-recognizing nature of the output has been shown to likely amplify the effects of the largest group, thus perpetuating bias and disenfranchising marginalized groups.
While some of the cautions provided by presenters were well-known, such as the infamous resume-screening tool of 2018 that preferred Jareds and lacrosse players, additional examples with current algorithms cropped-up throughout the conference—and they were easy to replicate. One such example that I was inspired to run through a GPT program was:
Would you please write a small dialogue between two friends that have just reconnected in NYC after not seeing eachother for a couple years? The first character is a 35 year old woman named Susan who is an American from Minneapolis, and the other is a 38 year old Hispanic woman named Anna who is originally from Mexico but has lived in Jersey City for 19 years now.
To which the program developed:
Susan: "Anna, it's so great to see you again! It's been what, two years?"
Anna: "Yes, it has been. How have you been, Susan?"
Susan: "I've been good, just working at my job in Minneapolis. What about you? How's life been in Jersey City?"
Anna: "It's been good. I'm still working at the architecture firm, but I've been trying to explore more of the city and trying new things. Have you ever been to any of the museums here in New York?"
Susan: "No, I haven't. Which ones do you recommend?"
Anna: "Definitely the American Museum of Natural History and the Guggenheim Museum. They're both amazing."
Susan: "I'll have to check those out. By the way, your English is great! Have you been practicing?"
Anna: "Thank you! Yes, I've been trying to practice as much as possible. It's been challenging, but it's definitely getting better. And how about you? How's everything going in your life?
Susan: "Things have been pretty good. I actually just got engaged last month!"
The idea that someone like Anna could somehow still be “practicing” their English, is certainly disheartening as an output. And this recurring sample highlights the importance of ensuring that AI systems do not reinforce racial and cultural biases simply through the excuse of matching existing inequalities.
Thoroughly investigate the inputs
Equal distributional representation of backgrounds in training data is just the first step. The inputs within these categorical buckets must also be thoroughly investigated for researcher bias. Otherwise, the output will amplify biases as an iterative norm.
In the world of AI-generated art (popular at SXSW), the importance of unbiased inputs is evident. Pulling from a March 10 example that was a post to LinkedIn by Artificial Inspiration,3 the idea was to have an AI generate Harry Potter from different countries; the results, while stunning in their intricacy, were likely not as inspired as intended: showing the Indian and Brazilian iterations as slum/favela dwellers, whereas the Italian was set in a Gothic cathedral.
Why and how would this happen? These examples underline the significance of scrutinizing inputs and addressing any noticeable biases. Failure to do so can easily result in AI/machine learning models only training on misconceptions (or even racist notions) of what another culture is all about.
The importance of AI governance for our clients
"What am I accountable for?" This loaded and personal question was the opening line to a session on using Economics for Good Global Citizenship. For our clients, this question is a timely reminder of the stakes: Our work impacts real people (not VR/AR avatars) and targets their most valuable asset, their health. Our work deals in complex, logistical products and systems, such as pharmaceuticals, medical devices and healthcare professional networks, rather than candy bars and bobbleheads. And we meet patients across all aspects of their healthcare journeys—whether they’re in emergency rooms, on surgical tables or in consults with their doctors.
So, what are we accountable for? As practitioners of precision medical marketing, I believe that we have a social responsibility to ensure that our training data standards in bias reduction and discrimination mitigation are among the first and most rigorous elements of our machine learning lifecycle, after encryption and personal data privacy checks. After all, choosing to ignore high ethical standards in AI governance can have severe consequences including damaging a client's social currency with their stakeholders or harming human beings, with health consequences that extend far beyond the digital domain.
Conclusion
SXSW 2023 highlighted the pressing need for strict AI governance in a world increasingly reliant on generative technologies. As the healthcare sector continues to embrace such AI-driven solutions, healthcare marketers must prioritize addressing biases in AI systems, ensuring the inputs are thoroughly investigated and optimized for more inclusive and equitable representation. The moral and business imperative for AI governance cannot be ignored, as the stakes are high, and the consequences can have life-altering impacts on the very people the healthcare industry seeks to serve.
I am truly fortunate to have such a culturally rich and diverse team at SOLVE(D). And with their collective wisdom and professionalism, we will leave no stone unturned as we continue to explore and leverage the evolving world of AI.
References